Syed Juned Ali
Univ.Ass. BSc MSc
Syed Juned Ali
- Email: syed.juned.ali@tuwien.ac.at
- Phone: +43-1-58801-194309
- Office: HG0211 (1040 Wien, Favoritenstrasse 11)
- About:
- Orcid: 0000-0003-1221-0278
- Keywords:
- Roles: PreDoc Researcher
Publications
CM2KGcloud – An open web-based platform to transform conceptual models into knowledge graphs
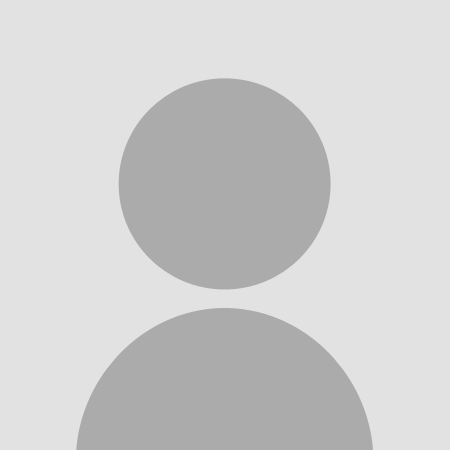
Keywords: Artificial intelligence, Cloud platform, Conceptual modeling, Knowledge graph, Model transformation, Model-driven engineering
Astract: Semantic processing of conceptual models is a focus of research for several years, bridging the disciplines of knowledge-based systems, conceptual modeling, and model-driven software engineering. With Knowledge Graphs, this research area gained momentum. In this paper, we introduce CM2KGcloud, a generic and extensible Web-based platform for transforming conceptual models into Knowledge Graphs. The platform can work on models created by state-of-the-art metamodeling platforms (e.g., EMF, Papyrus, ADOxx) and transforms models created with them into standardized Knowledge Graph representations like GraphML, RDF, and OWL. CM2KGcloud can be used as a service and can be integrated into software systems by its exposed API.
Smajevic, M., Ali, S. J., & Bork, D. (2024). CM2KGcloud – An open web-based platform to transform conceptual models into knowledge graphs. Science of Computer Programming, 231, Article 103007. https://doi.org/10.1016/j.scico.2023.103007
A Generic and Customizable Genetic Algorithms-Based Conceptual Model Modularization Framework
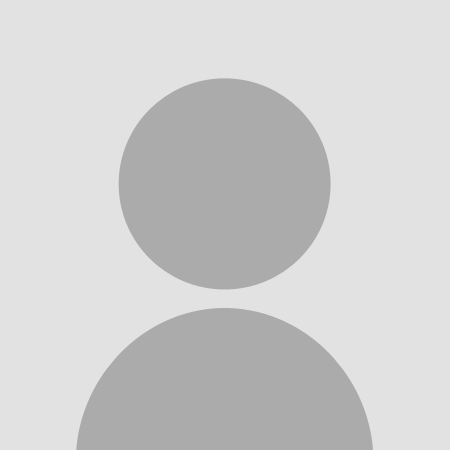
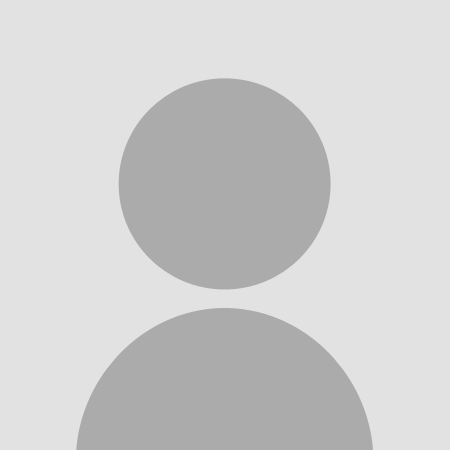
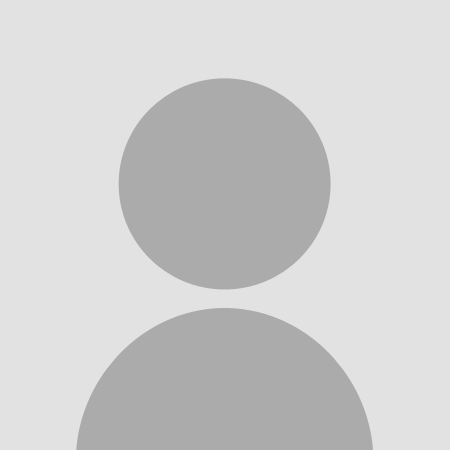
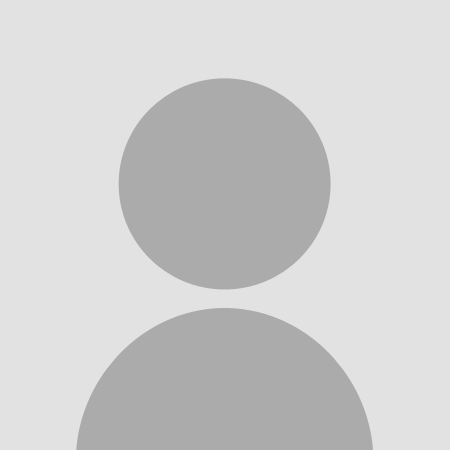
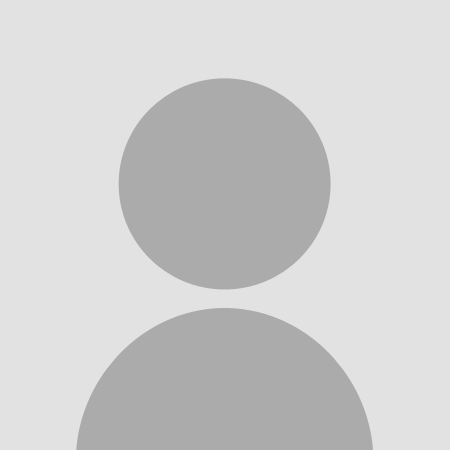
Keywords: Generic Framework, Genetic Algorithm, Modularization
Astract: Conceptual models need to be comprehensible and maintainable by humans to exploit their full value in faithfully representing a subject domain. Modularization, i.e. breaking down the monolithic model into smaller, comprehensible chunks has proven very valuable to maintain this value even for very large models. The quality of modularization however often depends on application-specific requirements, the domain, and the modeling language. A well-defined generic modularizing framework applicable to different modeling languages and requirements is lacking. In this paper, we present a customizable and generic multi-objective conceptual models modularization framework. The multi-objective aspect supports addressing heterogeneous requirements while the framework’s genericity supports modularization for arbitrary modeling languages and its customizability is provided by adopting the modularization configuration up to the level of using user-defined heuristics. Our approach applies genetic algorithms to search for a set of optimal solutions. In this paper, we present the details of our Generic Genetic Modularization Framework with a case study to show i) the feasibility of our approach by modularizing models from multiple modeling languages, ii) the customizability by using different objectives for the modularization quality, and, finally, iii) a comparative performance evaluation of our approach on a dataset of ER and ECore models.
Ali, S. J., Laranjo, J. M., & Bork, D. (2023). A Generic and Customizable Genetic Algorithms-Based Conceptual Model Modularization Framework. In H. Proper, L. Pufahl, D. Karastoyanova, M. van Sinderen, & J. Moreira (Eds.), Enterprise Design, Operations, and Computing : 27th International Conference, EDOC 2023, Groningen, The Netherlands, October 30 – November 3, 2023, Proceedings (pp. 39–57). Springer. https://doi.org/10.1007/978-3-031-46587-1_3
Keywords: Artificial intelligence, DMN, Enterprise modeling, Explainable AI, Machine learning, Model-driven engineering
Astract: The Decision Model and Notation (DMN) modeling language allows the precise specification of business decisions and business rules. DMN is readily understandable by business users involved in decision management. However, as the models get complex, the cognitive abilities of humans threaten manual maintainability and comprehensibility. Proper design of the decision logic thus requires comprehensive automated analysis of e.g., all possible cases the decision shall cover; correlations between inputs and outputs; and the importance of inputs for deriving the output. In the paper, the authors explore the mutual benefits of combining human-driven DMN decision modeling with the computational power of Artificial Intelligence for DMN model analysis and improved comprehension. The authors propose a model-driven approach that uses DMN models to generate Machine Learning (ML) training data and show, how the trained ML models can inform human decision modelers by means of superimposing the feature importance within the original DMN models. An evaluation with multiple real DMN models from an insurance company evaluates the feasibility and the utility of the approach.
Bork, D., Ali, S. J., & Dinev, G. M. (2023). AI-Enhanced Hybrid Decision Management. Business and Information Systems Engineering, 65(2), 179–199. https://doi.org/10.1007/s12599-023-00790-2
Knowledge graph-based generic modularisation of conceptual models
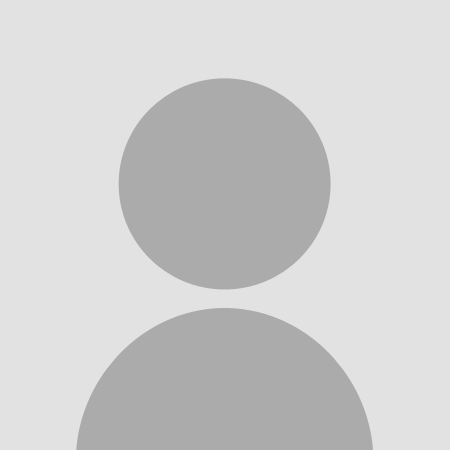
Keywords: conceptual modeling, knowledge graph, modulaisation, genetic algorithm, model-driven engineering
Astract: Conceptual models are an integral part of information systems. They abstract concrete instances to display and emphasise specific aspects. These models grow and over time lead to monolithic artefacts and increased complexity. Modularisation is a process and technique to reduce complexity and make conceptual models more comprehensible by decomposing monolithic artefacts into smaller, understandable modular units. However, automatically finding a good modularisation solution, especially for any conceptual model, is difficult.Currently, there is a lack of a framework that can automatically modularise any conceptual model without any manual operation, where users can easily control configurations and extend the framework. Using the same framework or tool for different conceptual models, e.g. UML or BPMN models, is impossible. Most automatic modularisation approaches focus on specific conceptual models.This thesis aims to develop a complete modularisation framework, called GGMF, which stands for Generic Genetic Modularisation Framework, with a user interface. Users can customise parameters and specify objective criteria for modularisation solutions. It embeds the CM2KG framework for transforming conceptual models into knowledge graphs. A genetic algorithm receives the knowledge graph as an input and determines modularisation solutions. The framework generates the solutions as modularised knowledge graphs. The framework is designed to be extensible. Users can define custom objectives for the fitness function and replace the genetic encoding and operators with their own definitions.Finally, the work is evaluated by focusing on the configuration aspects, the impact of parameter changes, the comprehensibility of modularisation solutions and comparison with state-of-the-art modularisation-related techniques.
Laranjo, J. M. (2023). Knowledge graph-based generic modularisation of conceptual models [Diploma Thesis, Technische Universität Wien]. reposiTUm. https://doi.org/10.34726/hss.2023.99585
Exploring Enterprise Architecture Knowledge Graphs in Archi: The EAKG Toolkit
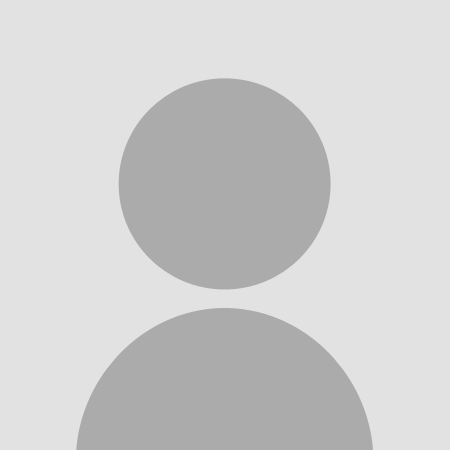
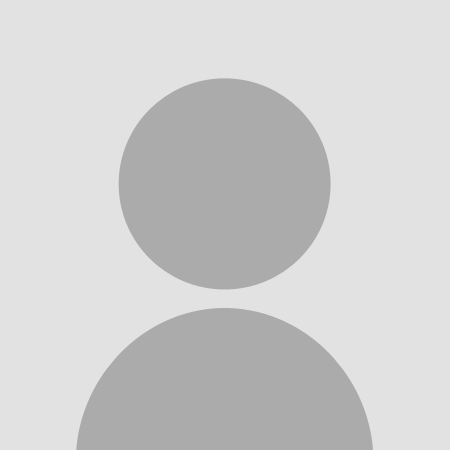
Keywords: Archi, ArchiMate, Enterprise architecture, Knowledge graph, Modeling tool
Astract: This paper presents the EAKG Toolkit that entails a new Knowledge Graph-based representation of enterprise architecture (EA) models and further enables reasoning on EA knowledge. Our developed EAKG Toolkit is unique in the sense that it i) transforms ArchiMate models into a KG representation – the Enterprise Architecture Knowledge Graph (EAKG), ii) visualizes the EAKG for interactive exploration, and iii) extends the EAKG with additional nodes and edges to visually represent detected EA smells.
Glaser, P.-L., Ali, S. J., Sallinger, E., & Bork, D. (2023). Exploring Enterprise Architecture Knowledge Graphs in Archi: The EAKG Toolkit. In Conference Proceedings: Enterprise Design, Operations, and Computing. EDOC 2022 Workshops (pp. 332–338). Springer. https://doi.org/10.1007/978-3-031-26886-1_21
Teaching
Model Engineering
Semester: 2024W; Nr: 188.923; Type: VU; Hours: 4.0; Language: English; View on TISSTeam
Business Informatics Group, TU Wien
Professors
Christian Huemer
Ao.Univ.Prof. Mag.rer.soc.oec.Dr.rer.soc.oec.
Dominik Bork
Associate Prof. Dipl.-Wirtsch.Inf.Univ.Dr.rer.pol.
Gerti Kappel
O.Univ.Prof.in Dipl.-Ing.inMag.a Dr.in techn.
Henderik Proper
Univ.Prof. PhDResearchers
Aleksandar Gavric
Univ.Ass. MEng. B.Eng.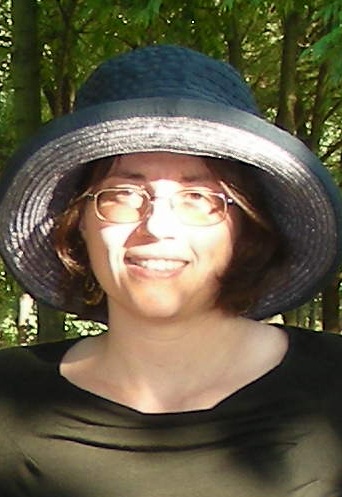
Galina Paskaleva
Projektass.in Dipl.-Ing.inDipl.-Ing.in BSc
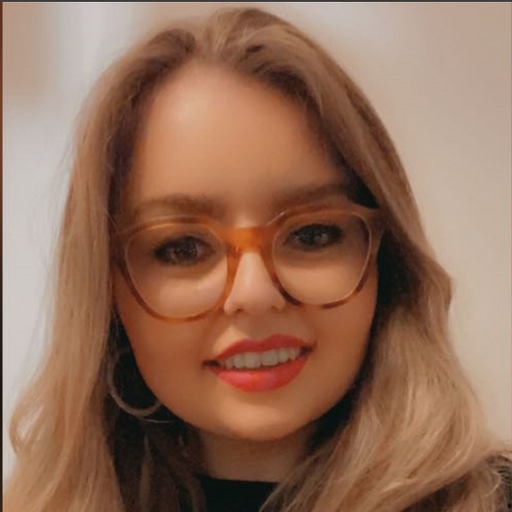
Marianne Schnellmann
Univ.Ass.in BSc MScMarion Murzek
Senior Lecturer Mag.a rer.soc.oec.Dr.in rer.soc.oec.
Marion Scholz
Senior Lecturer Dipl.-Ing.inMag.a rer.soc.oec.
Miki Zehetner
Univ.Ass. DI Bakk.rer.soc.oec. MScSyed Juned Ali
Univ.Ass. BSc MScStudent-Staff
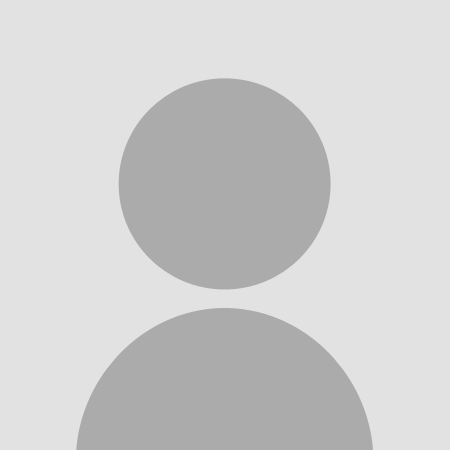
Florian Fankhauser
Projektass. Dipl.-Ing.Julia Smejkal
BSc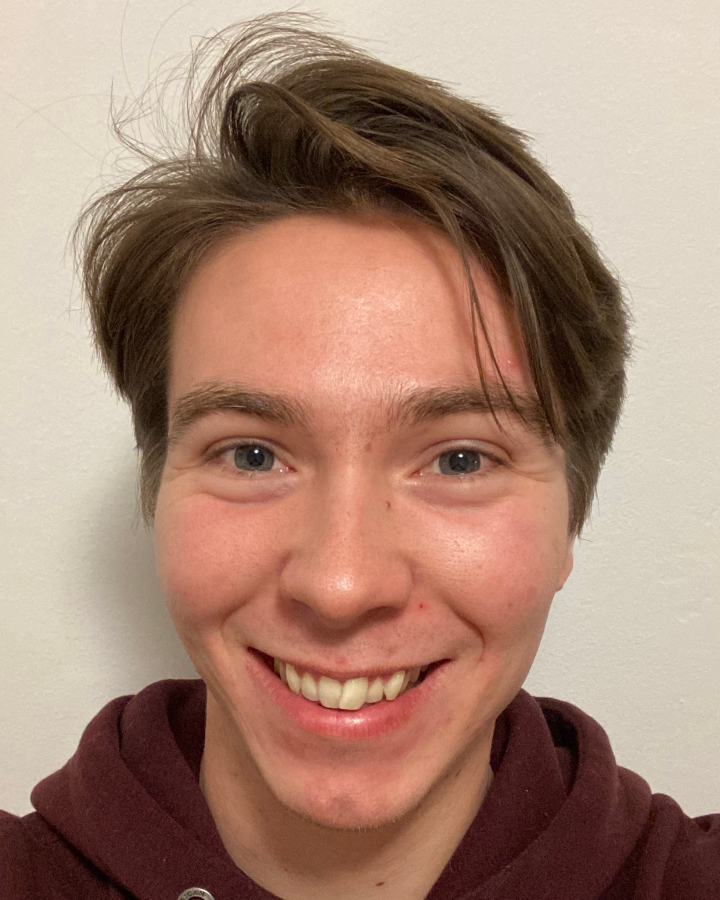