Dominik Bork
Associate Prof. Dipl.-Wirtsch.Inf.Univ.
Dr.rer.pol.
Dominik Bork
- Email: dominik.bork@tuwien.ac.at
- Phone: +43-1-58801-194308
- Office: HG0206 (1040 Wien, Favoritenstrasse 11)
- About:
Dominik Bork is working as an Assistant Professor for Business Systems Engineering at TU Wien since July 2020. Prior to moving to TU Wien, he worked as a Postdoc at the University of Vienna. He received his Diploma in Information Science and his PhD (Dr. rer. pol.) from the University of Bamberg where he primarily worked on multi-view enterprise modeling and metamodeling.
During his academic career, he was visiting researcher at and is up to date active collaborator with the University of Technology Sydney, the Instituto Tecnologico Autonomo de Mexico, the University of Pretoria, Stockholm University, and the Ecolé de Mines d’Albi.
Dominik Bork is elected domain expert of the Special Interest Group on Modelling Business Information Systems of the German Informatics Society (GI).
- Orcid: 0000-0001-8259-2297
- Keywords: Conceptual Modelling, UML, Model Engineering, Artificial intelligence, object oriented software design, Enterprise Architecture, Process Engineering
- Roles: Associate Professor
Publications
Introducing BIGUML: A Flexible Open-Source GLSP-Based Web Modeling Tool for UML
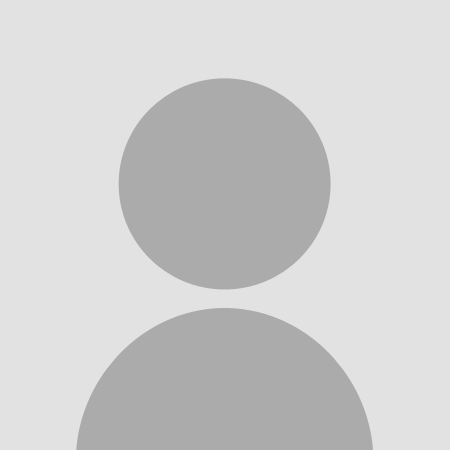
Metin, H., & Bork, D. (2023). Introducing BIGUML: A Flexible Open-Source GLSP-Based Web Modeling Tool for UML. In 2023 ACM/IEEE International Conference on Model Driven Engineering Languages and Systems Companion (MODELS-C) (pp. 40–44). IEEE. https://doi.org/10.1109/MODELS-C59198.2023.00016
On Developing and Operating GLSP-based Web Modeling Tools: Lessons Learned from BIGUML
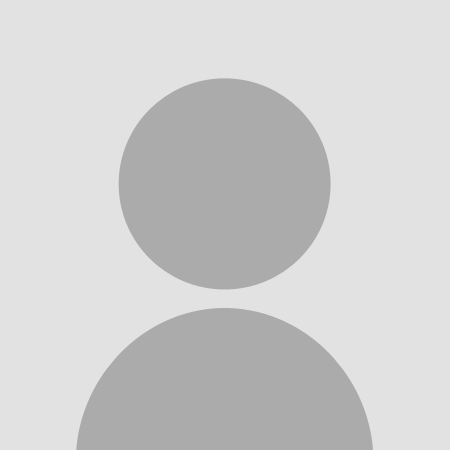
Keywords: Modeling tool, Knowledge engineering, Unified Modeling Language, Computer architecture, Model driven engineering, web modeling
Astract: The development of web-based modeling tools still poses significant challenges for developers. The Graphical Language Server Platform (GLSP) reduced some of these challenges by providing the necessary frameworks to efficiently create web modeling tools. However, more knowledge and experience are required regarding developing GLSP-based web modeling tools. This paper discusses the challenges and lessons learned after working with GLSP and realizing several GLSP-based modeling tools. More concretely, experiences, concepts, steps to be followed to develop and operate a GLSP-based web modeling tool, and the advantages and disadvantages of working with GLSP are discussed. As a proof of concept, we will report on the realization of a GLSP-based UML editor called BIGUML. Through BIGUML, we show that our procedure and the reference architecture we developed resulted in a scalable and flexible GLSP-based web modeling tool. The lessons learned, the procedural approach, the reference architecture, and the critical reflection on the challenges and opportunities of using GLSP provide valuable insights to the community and shall ease the decision of whether or not to use GLSP for future tool development projects.
Metin, H., & Bork, D. (2023). On Developing and Operating GLSP-based Web Modeling Tools: Lessons Learned from BIGUML. In 2023 ACM/IEEE 26th International Conference on Model Driven Engineering Languages and Systems (MODELS) (pp. 129–139). IEEE. https://doi.org/10.1109/MODELS58315.2023.00031
Encoding Conceptual Models for Machine Learning: A Systematic Review
Keywords: Conceptual Modeling, Analytical models, Systematics, Machine Learning algorithms, Bibliographies, Semantics, Machine Learning
Astract: Conceptual models are essential in Software and Information Systems Engineering to meet many purposes since they explicitly represent the subject domains. Machine Learning (ML) approaches have recently been used in conceptual modeling to realize, among others, intelligent modeling assistance, model transformation, and metamodel classification. These works en-code models in various ways, making the encoded models suitable for applying ML algorithms. The encodings capture the models' structure and/or semantics, making this information available to the ML model during training. Therefore, the choice of the encoding for any ML-driven task is crucial for the ML model to learn the relevant contextual information. In this paper, we report findings from a systematic literature review which yields insights into the current research in machine learning for conceptual modeling (ML4CM). The review focuses on the various encodings used in existing ML4CM solutions and provides insights into i) which are the information sources, ii) how is the conceptual model's structure and/or semantics encoded, iii) why is the model encoded, i.e., for which conceptual modeling task and, iv) which ML algorithms are applied. The results aim to structure the state of the art in encoding conceptual models for ML.
Ali, S. J., Gavric, A., Proper, H., & Bork, D. (2023). Encoding Conceptual Models for Machine Learning: A Systematic Review. In 2023 ACM/IEEE International Conference on Model Driven Engineering Languages and Systems Companion (MODELS-C) (pp. 562–570). IEEE. https://doi.org/10.1109/MODELS-C59198.2023.00094
Keywords: Digital Twin, Knowledge engineering, Model driven engineering, circular economy, sustainability
Astract: The next generation of engineered systems ought to be more sustainable. In this context, Digital Twins play a crucial role as key enablers of sustainability ambitions in systems engineering. However, as a specific class of engineered systems, Digital Twins themselves must adopt sustainability principles to avoid defeating their purpose in fostering sustainability. In this proposal, we focus on the technical sustainability of Digital Twins, enabled by their evolution. We propose an initial taxonomy we believe will support systematic Digital Twin evolution mecha-nisms and draw links to similar taxonomies of Physical Twins.
David, I., & Bork, D. (2023). Towards a Taxonomy of Digital Twin Evolution for Technical Sustainability. In 2023 ACM/IEEE International Conference on Model Driven Engineering Languages and Systems Companion (MODELS-C) (pp. 934–938). IEEE. https://doi.org/10.1109/MODELS-C59198.2023.00147
Enterprise Modeling for Machine Learning: Case-Based Analysis and Initial Framework Proposal
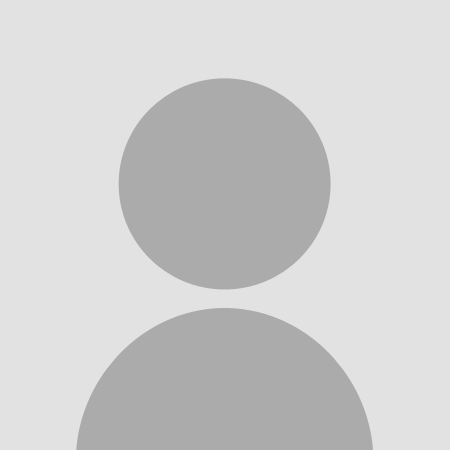
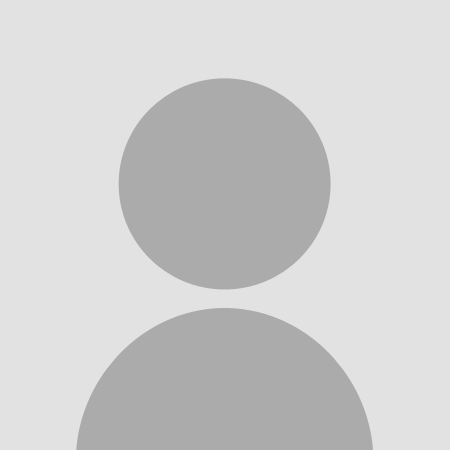
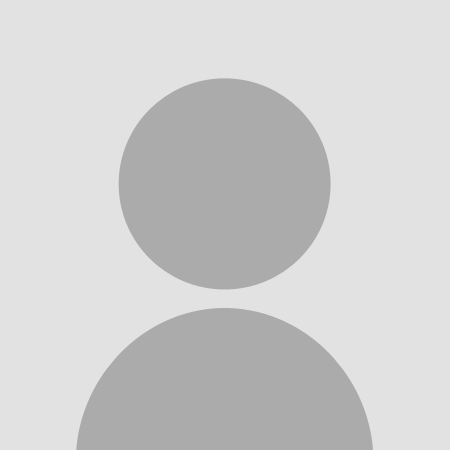
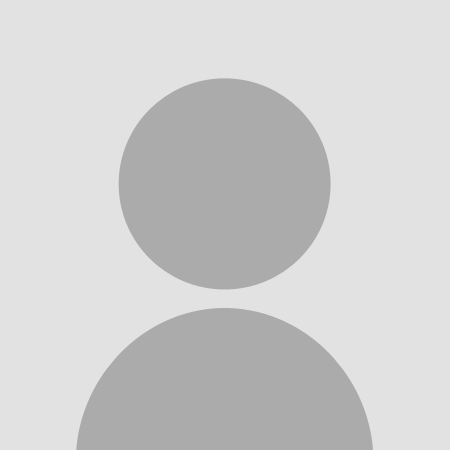
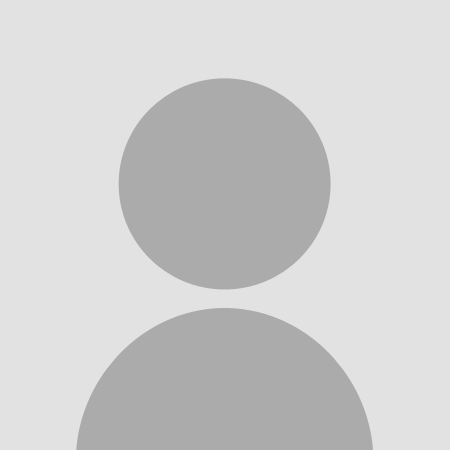
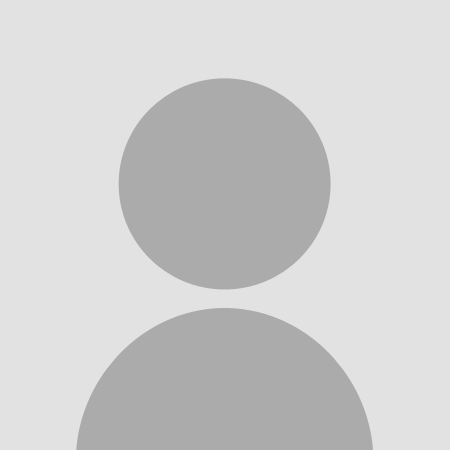
Keywords: Artificial intelligence, Conceptual modeling, Enterprise modeling, Machine learning, Model-driven engineering
Astract: Artificial Intelligence (AI) continuously paves its way into even the most traditional business domains. This particularly applies to data-driven AI, like machine learning (ML). Several data-driven approaches like CRISP-DM and KKD exist that help develop and engineer new ML-enhanced solutions. A new breed of approaches, often called canvas-driven or visual ideation approaches, extend the scope by a perspective on the business value an ML-enhanced solution shall enable. In this paper, we reflect on two recent ML projects. We show that the data-driven and canvas-driven approaches cover only some necessary information for developing and operating ML-enhanced solutions. Consequently, we propose to put ML into an enterprise context for which we sketch a first framework and spark the role enterprise modeling can play.
Bork, D., Papapetrou, P., & Zdravkovic, J. (2023). Enterprise Modeling for Machine Learning: Case-Based Analysis and Initial Framework Proposal. In S. Nurcan, A. L. Opdahl, H. Mouratidis, & A. Tsohou (Eds.), Research Challenges in Information Science: Information Science and the Connected World : 17th International Conference, RCIS 2023, Corfu, Greece, May 23–26, 2023, Proceedings (pp. 518–525). Springer. https://doi.org/10.1007/978-3-031-33080-3_33
Teaching
Seminar for Master Students in Software Engineering & Internet Computing
Semester: 2024W; Nr: 180.777; Type: SE; Hours: 1.0; Language: English; View on TISSResearch Seminar
Semester: 2024W; Nr: 188.446; Type: SE; Hours: 2.0; Language: if required in English; View on TISSLiterature Seminar for PhD Students
Semester: 2024W; Nr: 188.512; Type: SE; Hours: 2.0; Language: German; View on TISSModel Engineering
Semester: 2024W; Nr: 188.923; Type: VU; Hours: 4.0; Language: English; View on TISSBachelor Thesis for Informatics and Business Informatics
Semester: 2024W; Nr: 188.926; Type: PR; Hours: 5.0; Language: if required in English; View on TISSSoftware Engineering
Semester: 2024W; Nr: 194.020; Type: VU; Hours: 4.0; Language: German; View on TISSProject in Computer Science 1
Semester: 2024W; Nr: 194.145; Type: PR; Hours: 4.0; Language: if required in English; View on TISSProjects
JSON-basierte, web-natives Modellierungsframework für Model-Diffing
Name: JSONVerse; Title: JSON-basierte, web-natives Modellierungsframework für Model-Diffing; Begins On: 2024-07-01; Ends On: 2025-01-31; Context: Austrian Research Promotion Agency (FFG); View Project WebsiteTowards Low-Code Business App Development - ER2CDS
Name: ER2CDS; Title: Towards Low-Code Business App Development - ER2CDS; Begins On: 2024-01-01; Ends On: 2024-12-31; Context: valantic Business Technology & Transformatio GmbH; View Project WebsiteAutomatisiertes End-to-End-Testen von Cloud-basierten Modellierungswerkzeugen
Name: InnoScheckEclipsesource23; Title: Automatisiertes End-to-End-Testen von Cloud-basierten Modellierungswerkzeugen; Begins On: 2023-05-01; Ends On: 2024-04-30; Context: Austrian Research Promotion Agency (FFG); View Project WebsiteDiplomarbeitsbetreuung AI Readiness Assessment
Name: DA-EFS; Title: Diplomarbeitsbetreuung AI Readiness Assessment; Begins On: 2023-01-24; Ends On: 2024-01-23; Context: EFS Unternehmensberatung GesmbH; View Project WebsiteMFP 4.2 Advanced Analytics for Smart Manufacturing
Name: MFP 4.2; Title: MFP 4.2 Advanced Analytics for Smart Manufacturing; Begins On: 2022-10-01; Ends On: 2023-09-30; Context: CDP Center for Digital Production G; View Project WebsiteDigital Platform Enterprise
Name: DEMO; Title: Digital Platform Enterprise; Begins On: 2022-01-01; Ends On: 2024-12-31; Context: European Commission; View Project WebsiteTeam
Business Informatics Group, TU Wien
Professors
Christian Huemer
Ao.Univ.Prof. Mag.rer.soc.oec.Dr.rer.soc.oec.
Dominik Bork
Associate Prof. Dipl.-Wirtsch.Inf.Univ.Dr.rer.pol.
Gerti Kappel
O.Univ.Prof.in Dipl.-Ing.inMag.a Dr.in techn.
Henderik Proper
Univ.Prof. PhDResearchers
Aleksandar Gavric
Univ.Ass. MEng. B.Eng.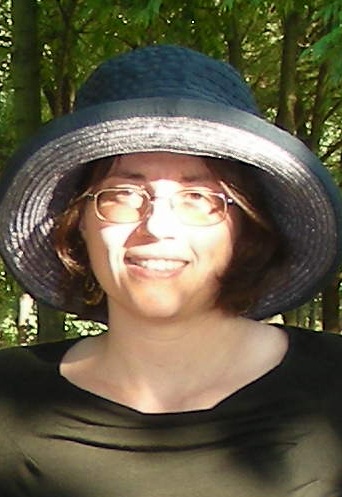
Galina Paskaleva
Projektass.in Dipl.-Ing.inDipl.-Ing.in BSc
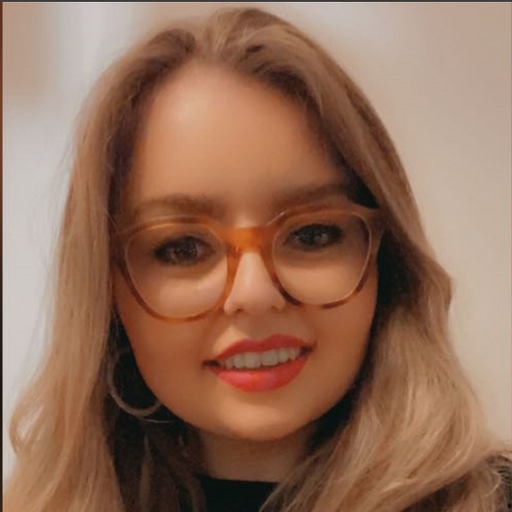
Marianne Schnellmann
Univ.Ass.in BSc MScMarion Murzek
Senior Lecturer Mag.a rer.soc.oec.Dr.in rer.soc.oec.
Marion Scholz
Senior Lecturer Dipl.-Ing.inMag.a rer.soc.oec.
Miki Zehetner
Univ.Ass. DI Bakk.rer.soc.oec. MScSyed Juned Ali
Univ.Ass. BSc MScStudent-Staff
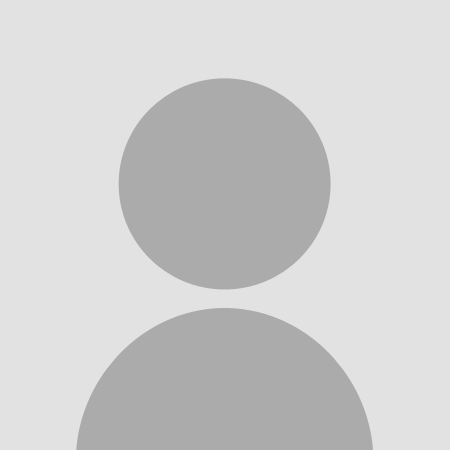
Florian Fankhauser
Projektass. Dipl.-Ing.Julia Smejkal
BSc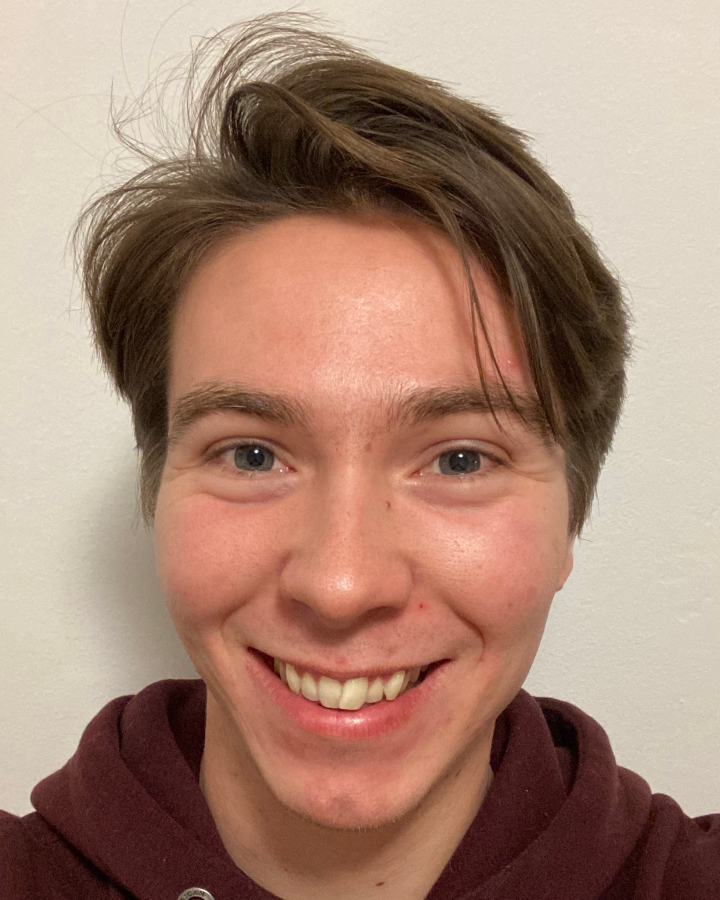